Algorithm may help diagnose PH when echocardiography uncertain
Too many without disease but lacking confirmation sent for invasive tests
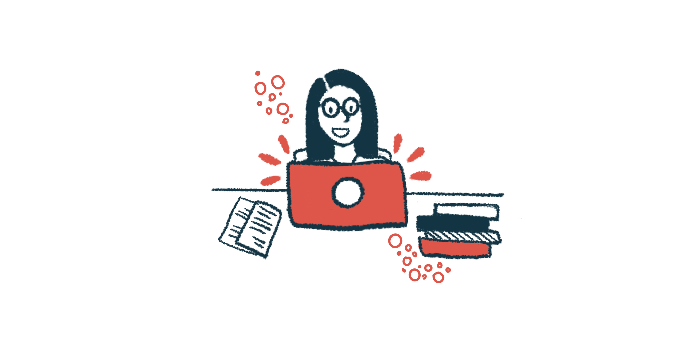
An algorithm based on artificial intelligence (AI) and machine learning may help in diagnosing pulmonary hypertension (PH), a new study shows.
Its findings are particularly important for people suspected of having PH but whose disease remains uncertain using echocardiography, a noninvasive imaging method to examine heart structure and function. These individuals, who may not have PH, often are referred for further evaluations requiring invasive tests.
“Our model may allow physicians to make a more accurate and reproducible echocardiographic estimation of normal vs elevated pulmonary pressures with greater efficiency and reduce referral for invasive testing,” the researchers wrote in “Machine Learning for Diagnosis of Pulmonary Hypertension by Echocardiography,” a study published in Mayo Clinical Proceedings.
Noninvasive echocardiography is among initial diagnostic PH tests
“The study … is timely, clinically relevant, and innovative in its application of machine learning in diagnosing pulmonary hypertension,” wrote Karl A. Nath, MD, the journal’s editor-in-chief, in its monthly highlights.
PH is marked by the narrowing of the pulmonary arteries, the blood vessels that transport blood through the lungs, causing high blood pressure and making the heart work harder.
Diagnosing PH can require multiple tests, including echocardiography. This technique estimates pulmonary artery pressure (PAP) by assessing the tricuspid valve, which controls blood flow from the heart’s right atrium to the right ventricle. Tricuspid regurgitation frequently is associated with PH, and it occurs when the valve does not close properly and blood flows backwards.
When artery pressure is elevated, diagnosis is confirmed by an invasive technique called right heart catheterization (RHC), in which a flexible tube, a catheter, is passed into the right side of the heart and into the pulmonary arteries to measure PAP.
People with normal findings on echocardiography often do not require further assessments. However, in more than a third of PH patients, PAP cannot be determined by echocardiography, making RHC necessary, the researchers noted.
A number of small studies suggest that other measures of heart function and blood tests are associated with PH. But no computer-based models exist to evaluate “with high sensitivity” the risk of PH when tricuspid regurgitation is unavailable.
Researchers at Mayo Clinic developed a predictive algorithm based on AI and machine learning that uses data obtained from echocardiography. To develop the algorithm, they analyzed data from 7,853 patients (mean age 64, 56% men) who underwent echocardiography and right heart catheterization at the Mayo Clinic in Minnesota between January 2012 and December 2019.
A total of 6,323 patients (81%) were diagnosed with PH, following the criteria proposed in 2018 at the 6th World Symposium on Pulmonary Hypertension: a mean PAP greater than 20 millimeters of mercury (mm Hg).
The heart’s right and left ventricles also had an abnormal size in 68% and 32% of the patients, respectively.
But for another 2,007 people (26%), tricuspid regurgitation velocity could not be measured.
Patients’ data were randomly divided into three groups: one for training the algorithm (5,024 people, 64%), another for validation (1,275 patients, 16%), and the third for testing (1,554 people, 20%).
The final model had 19 features, including patients’ age, sex, body mass index (a measure of body fat), estimated PAP, the functioning of the tricuspid valve, and heart rate.
Researchers first calculated the area under the receiver operating characteristic curve (AUC). This measure tells how well a given parameter can differentiate between two groups (i.e., PH or not). AUC values range from 0.5 to 1, with higher numbers indicating a better ability to differentiate.
Based on the validation data, an AUC threshold of 0.65 or higher had a sensitivity of 90% in identifying correctly those with the disease.
This threshold then was selected to assess the model’s performance in the testing datasets. Results showed that the model had high discrimination for the detection of PH with an accuracy of 82% and a sensitivity of 88%.
Model had good positive predictive value, but less effective in disease’s absence
In addition, the model’s positive predictive value was 89%, which refers to the percentage of patients who truly have PH after screening positive. The negative predictive value was 54%, meaning that 54% of cases truly did not have PH after a negative test.
“We hypothesize that future research that combines echocardiography (derived features and image processing techniques) with electrocardiographic [EKG] analysis may be required to come closer to the goal of 90% negative predictive value. The importance of this accomplishment will be that RHC to determine PH may become unnecessary in patients who do not have PH,” the researchers wrote.
The top five features supporting the model’s performance were estimated right atrial pressure, atrial fibrillation or flutter (an irregular or rapid beating of the heart’s atria), impression of the right ventricle’s normal function, body mass index, and heart rate.
Finally, in a group of 412 patients (27%) whose tricuspid regurgitation could not be measured, AUC was 0.785
An “AI assessment of echocardiographic data appears promising for estimating the presence of PH even when the [tricuspid regurgitation] velocity cannot be measured,” the team concluded, noting that larger validation studies are needed.
Among the study’s limitations, the researchers noted the nearly 80% of participants whose mean PAP was higher than 20 mm Hg, “which is a value much higher than one would expect under a more general screening setting,” and may have led to overtraining or “overfitting the model to the data.”
Study findings “demonstrate that the application of machine learning to the transthoracic echocardiogram enables the detection of pulmonary hypertension and may be used to identify patients with a low likelihood of pulmonary hypertension,” Nath wrote.