Corvista point-of-care device good at detecting heart failure: Study
System uses machine learning to predict PH in patients in noninvasive way
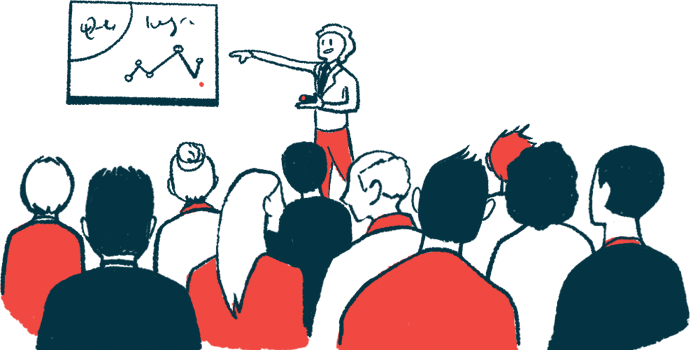
Corvista Health’s point-of-care device, which can be used to predict pulmonary hypertension in a noninvasive way, also appears to work well at identifying heart failure, accurately detecting patients who have it while ruling it out in others, a study found.
Using machine learning to decode data from patients, the Corvista System accurately detected elevated pulmonary capillary wedge pressure, or PCWP — an indirect measure of pressure in the heart’s left top chamber, which receives oxygen-rich blood from the lungs.
In the study, researchers at Corvista calculated that the diagnostic odds ratio of the machine learning algorithm was 29, meaning a patient with heart failure was 29 times more likely to test positive than a patient without heart failure.
“The new data provide preliminary results for the feasibility of our noninvasive machine learning-based test to detect elevated PCWP with a high level of accuracy,” Charles R. Bridges, MD, Corvista’s executive vice president and chief scientific officer, said in a company press release.
Bridges presented these data in a poster at the American College of Cardiology’s Annual Scientific Session, held late last month in Chicago. The poster was titled “Point-of-Care Testing for Pulmonary Capillary Wedge Pressure Elevation Using Machine Learning on Non-invasive Signals.”
Symptoms of PH, heart failure overlap, complicating diagnosis
Pulmonary hypertension occurs when blood pressure in the vessels that carry blood from the heart to the lungs becomes abnormally high. This makes it harder for the heart to pump enough blood to the lungs, leading to symptoms such as shortness of breath. If left untreated, it can result in heart failure.
Diagnosing pulmonary hypertension and heart failure can be difficult because many of their symptoms overlap with those of other conditions. The process is often lengthy and involves several different tests.
With the Corvista System, signals naturally emitted by the heart are collected for 3.5 minutes while the person is at rest. A machine learning algorithm analyzes the data in the cloud — a remote data processing service — making the results quickly available to doctors through a dedicated web portal.
In a previous study, the company showed that its machine learning algorithm performed comparably to transthoracic echocardiography — a standard diagnostic test to measure blood flow through the heart — in predicting pulmonary hypertension in patients with new-onset symptoms of cardiovascular disease.
Bridges noted, however, that “although echocardiography is an excellent modality for estimating left ventricular ejection fraction (EF), its sensitivity for diagnosing diastolic dysfunction is rather abysmal.”
EF refers to how much blood the heart’s left bottom chamber can pump. Diastolic dysfunction, meanwhile, is the heart’s inability to relax and fill with blood between heartbeats, which, if impaired, can lead to heart failure with preserved EF, or HFpEF.
While it accounts for about half of all cases of heart failure, “the underdiagnosis of HFpEF is arguably the largest unmet need in cardiovascular medicine today,” according to Bridges, who noted that it represents “approximately $25 billion in direct costs to the U.S. healthcare system annually.”
Algorithm ‘promising’ for development of point-of-care test for PCWP
The new study involved 283 patients, according to the poster’s abstract.
According to the researchers, the machine learning algorithm “achieved a clinically relevant performance in the identification of [patients] with elevated PCWP.”
Its sensitivity was 90%, meaning it correctly identified 9 of every 10 patients with heart failure. Its specificity was 76%, meaning it correctly ruled out slightly more than three-quarters of patients without heart failure.
It is critically important to differentiate subtypes of pulmonary hypertension which have distinct often lifesaving guideline-directed treatments.
A total of 247 features collected from data were found to help differentiate patients with elevated PCWP (greater than 18 mmHg) from those with a normal measurement (equal to or less than 18 mm Hg). The data were measured using a procedure called invasive right heart catheterization.
The algorithm also showed a positive likelihood ratio of 3.8 and a negative likelihood ratio of 0.13. This means that if a patient tests positive, they are more likely to have heart failure; if a patient tests negative, they are unlikely to have heart failure.
It performed well across different groups, such as by sex and age, according to the researchers.
Bridges noted that pulmonary hypertension “is also vastly underdiagnosed,” saying “it is critically important to differentiate subtypes of pulmonary hypertension which have distinct often lifesaving guideline-directed treatments.” Based on guidelines, PCWP is required to differentiate subtypes of pulmonary hypertension.
The researchers suggest their algorithm “is promising for the future development of a noninvasive, point-of-care test for PCWP.”
Per the team, “estimating PCWP would facilitate earlier diagnosis and appropriate treatment of various conditions, including heart failure and [pulmonary hypertension].”