Actelion, Owkin Partner on Machine Learning to Improve Clinical Trials
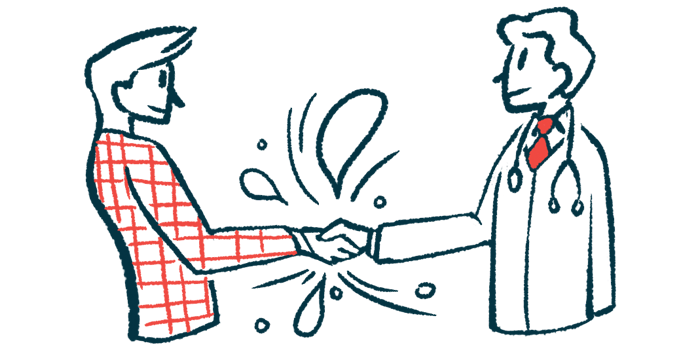
A joint effort between the startup Owkin and Actelion Pharmaceuticals, one of the Janssen companies, will seek to develop machine learning techniques that could improve how clinical trials are designed, and how the results are analyzed.
While the two companies will work to create tools that can be applied in trials for any disease, the first part of the project is specifically focused on pulmonary arterial hypertension (PAH).
“We’re thrilled to embark on this project to demonstrate how imperative machine learning methodologies are to improve clinical trial design and evaluation,” Gilles Wainrib, Owkin’s co-founder and chief science officer, said in a press release.
Clinical trials are needed for figuring out whether or not an experimental therapy is effective, and safe for patients. Pharmaceutical companies must complete multiple trials before seeking regulatory approval to market new medicines.
However, running clinical trials in the real world requires navigating a host of barriers and challenges.
This is especially true for a rare disease like pulmonary hypertension, for which the number of people who are eligible, willing, and able to participate in clinical trials is limited. Few available participants mean that trials tend to be small. In a statistical sense, smaller studies have less power to detect a significant effect from an investigational treatment.
One strategy that can be used to get around this limitation is to use external control groups.
In most clinical trials, participants are divided into two or more groups that get different treatments; for example, it’s common for some trial participants to be given the active medication, while others are given an inactive placebo. This creates an experimental group — the patients given active treatment — whose results can be compared against the control group, or those on the placebo.
An external control group, as the name suggests, involves using a group outside of the clinical trial for comparison. For instance, a given trial might give all participants an investigational treatment, then compare the results to patients who received a placebo or a different treatment in a separate study.
While helpful in settings with limited participants, this strategy has obvious drawbacks: the whole point of a clinical trial is that all participants are being cared for basically the same way, except for the specific differences being investigated in the trial. With an external control group, it’s possible that other differences could influence the results, and this may not be obvious when data are analyzed.
The goal of the venture between Owkin and Actelion (now Janssen Pulmonary Hypertension, part of the Johnson & Johnson pharmaceutical companies) is to use a technique called double/debiased machine learning to account for these confounding variables — the factors that are inconsistent in different trials.
“The aim of this initial project with Janssen’s R&D Data Science team is to investigate innovative machine learning-based methods for the estimation of treatment effect in clinical trials involving real-world data sources,” according to Owkin.
This technique may be a way of achieving enough statistical power to draw reliable conclusions in clinical trials with external control groups, the companies said.
The first project for the partners will focus on PAH, for which there remains no cure. PAH is a rare, progressive disease in which the pressure in the blood vessels of the lungs is elevated, resulting in stress on the heart. Owkin researchers note that early diagnosis and treatment are crucial for improving life expectancy in PAH.
Ultimately, the companies’ collaboration “has potential to help bring safe and effective drugs to patients faster,” Wainrib said.